Projects
Interactive Learning with Smart Pricing for Optimal and Stable Allocations in Markets
We study the joint effect of pricing and recommendations in markets such as AirBnB, Uber, Doordash, Amazon, eBay, etc. We develop an efficient algorithm to minimize customer dissatisfaction and market instability in a repeated learning setting by integrating techniqes such as collaborative filtering, explore and exploit, and bidding protocols for optimal resource allocation.
Collaborators: YE Eringbas, K Ramchandran (UC Berkeley)
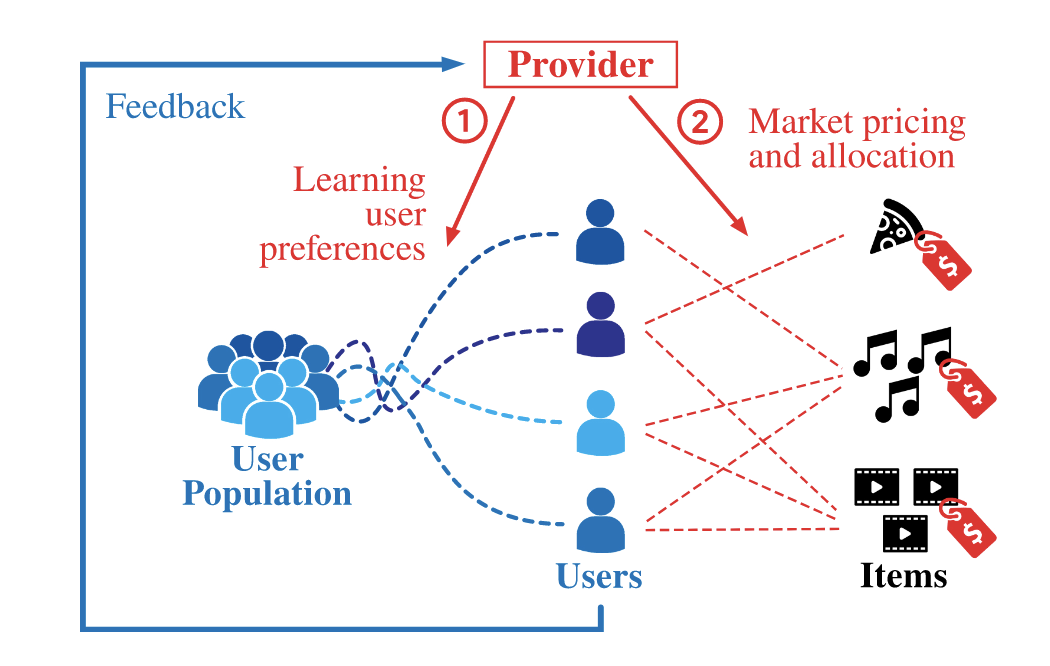
Building Enhanced Markets using AI
How to design assistive AI agents that learn individual user preferences, find top recommended actions out of several options for their user, bid opportunistically on behalf of the user in competing markets, be aware of the changing market conditions, and learn efficiently from past interactions? In this project we study these and other similar compelling questions.
Collaborators: YE Eringbas, L Butler, K Ramchandran (UC Berkeley)
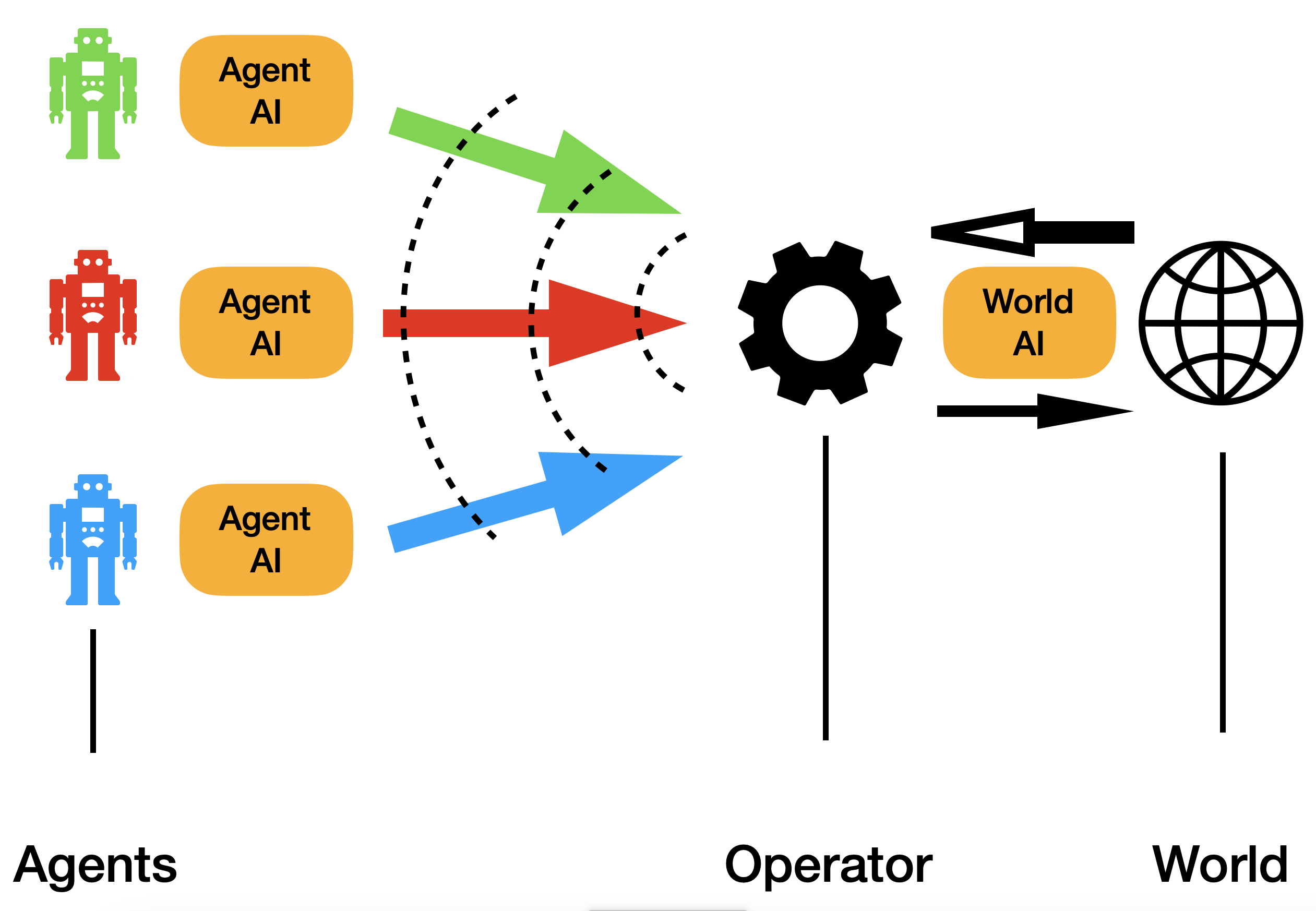
AI for climate change cooperation competition
RICE-N simulation.
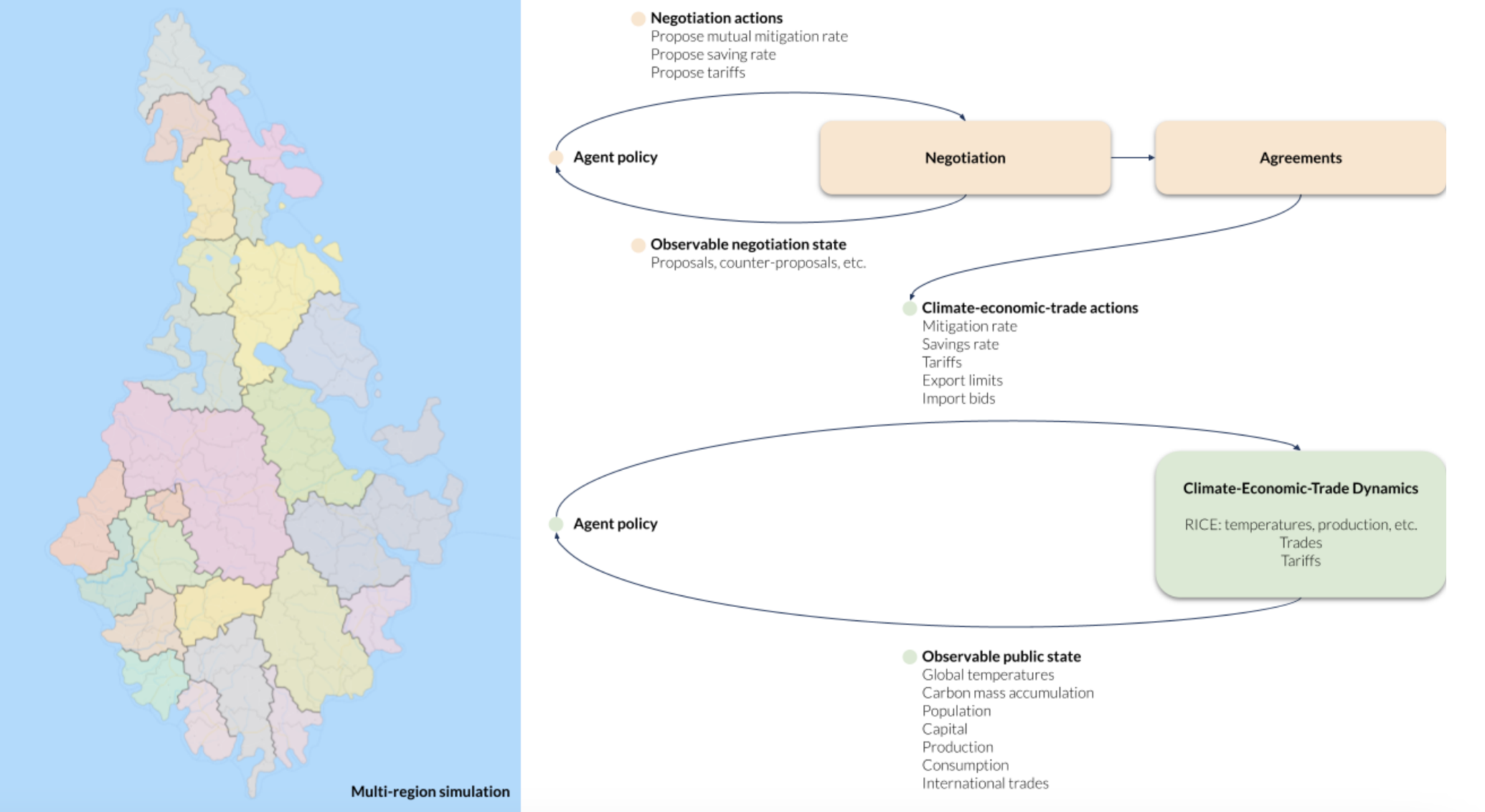
Reinforcement Learning for Economic Policymaking
Deep reinforcement learning has shown promising results in games with a limited number of agents such as Chess, Go, Poker, etc. We develop methods to go beyond few agent settings and apply it to microeconomic simulations for finding general equilibria and optimal agent policies.
Collaborators: Stephan Zheng, Sunil Srinivasa, Stefano Ermon (Salesforce Research)
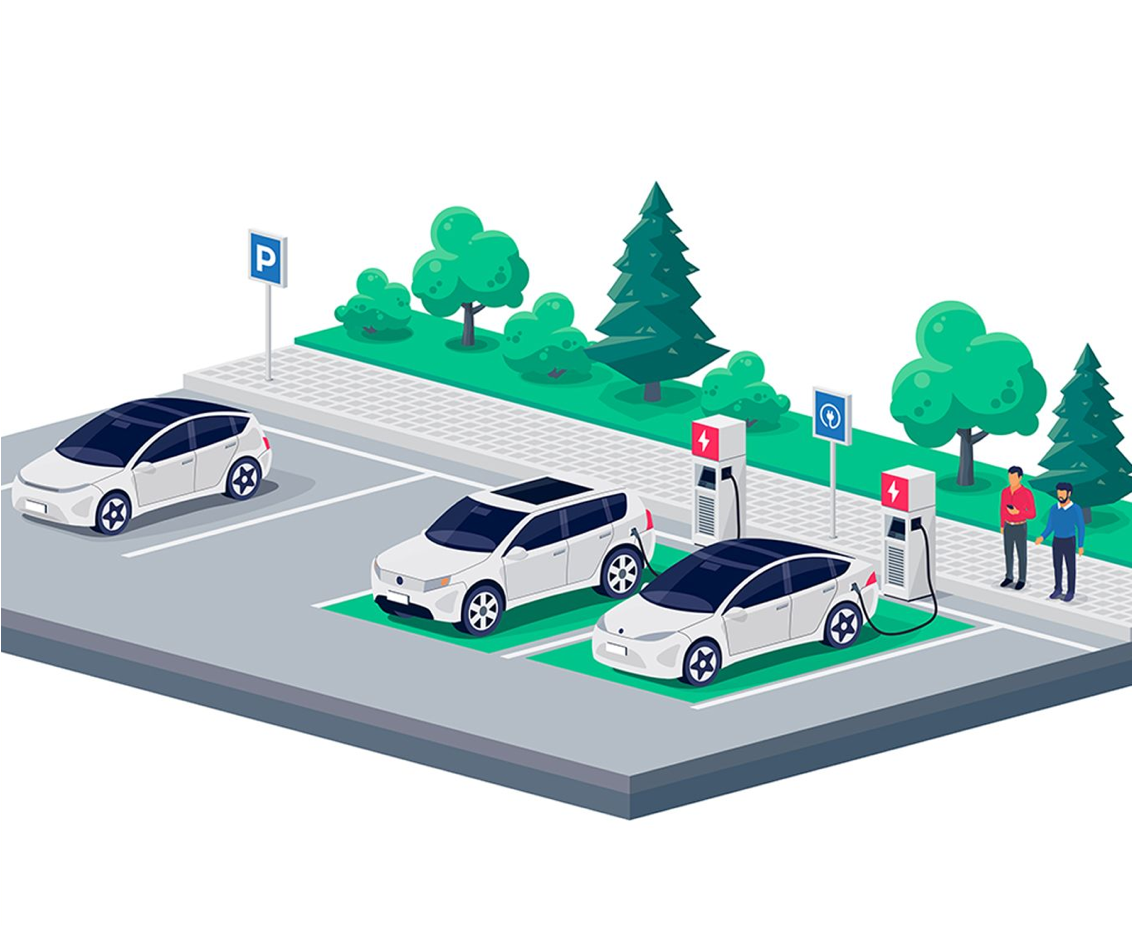
Behavioral Network Economics
As part of my PhD thesis, I focused on the role of behavioral models from psychology and decision theory (such as Cumulative Prospect Theory) in building efficient commercial systems that interact with humans.
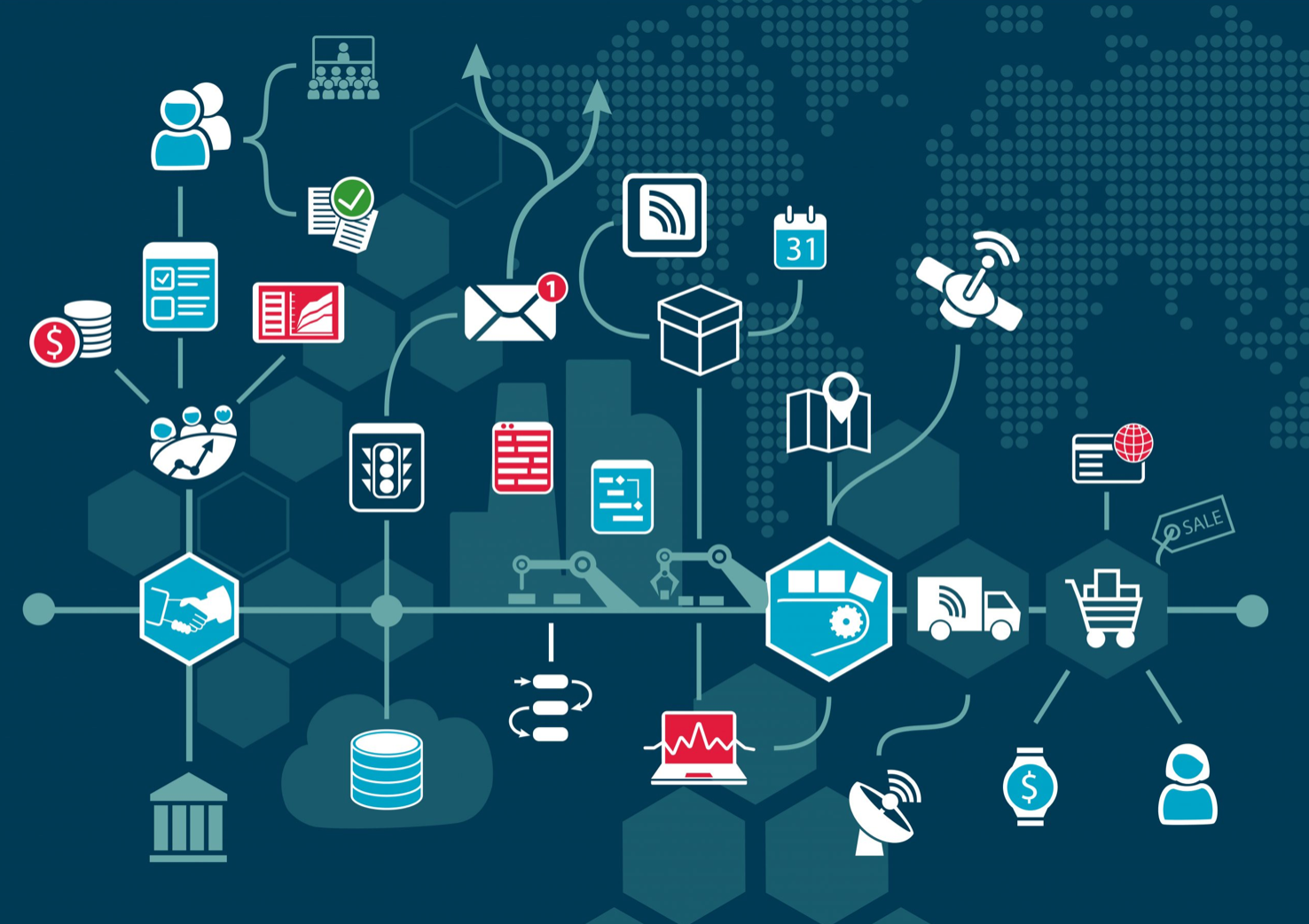
Online convex optimization and games
No-regret learning based agents in repeated games.
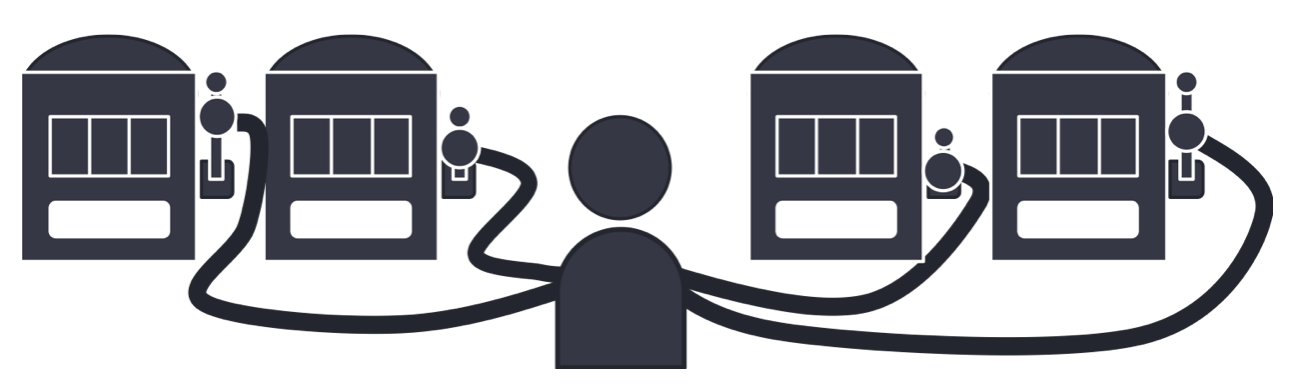
Session Aware Search Recommendations
Extreme multi-label classification.
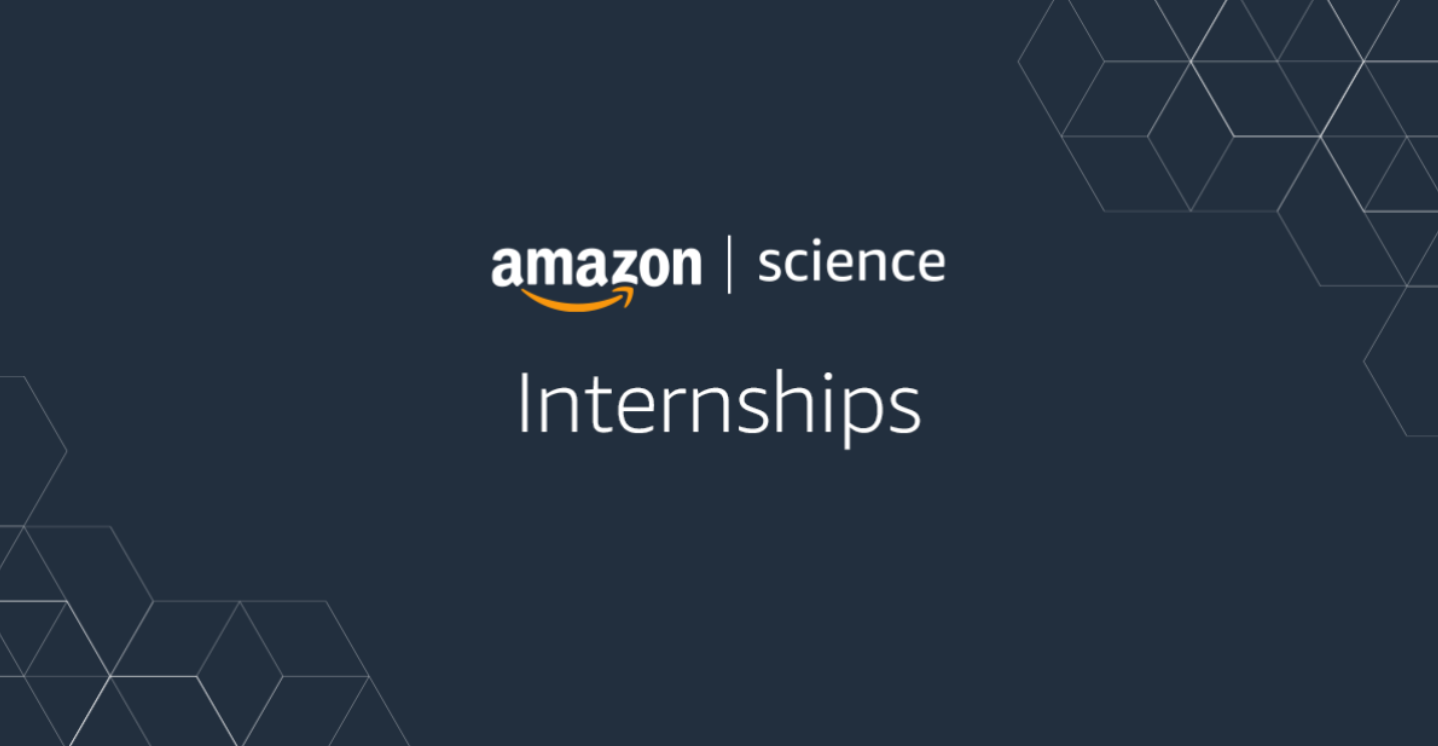
Lottery-based optimal resource allocation
We develop the theory behind lotteries to incentivize people (eg. flight upgrades and middle-seat lotteries) and invent a lottery-based version of the TCP/IP protocol to design optimal lotteries.
Collaborators: V Anantharam (UC Berkeley)
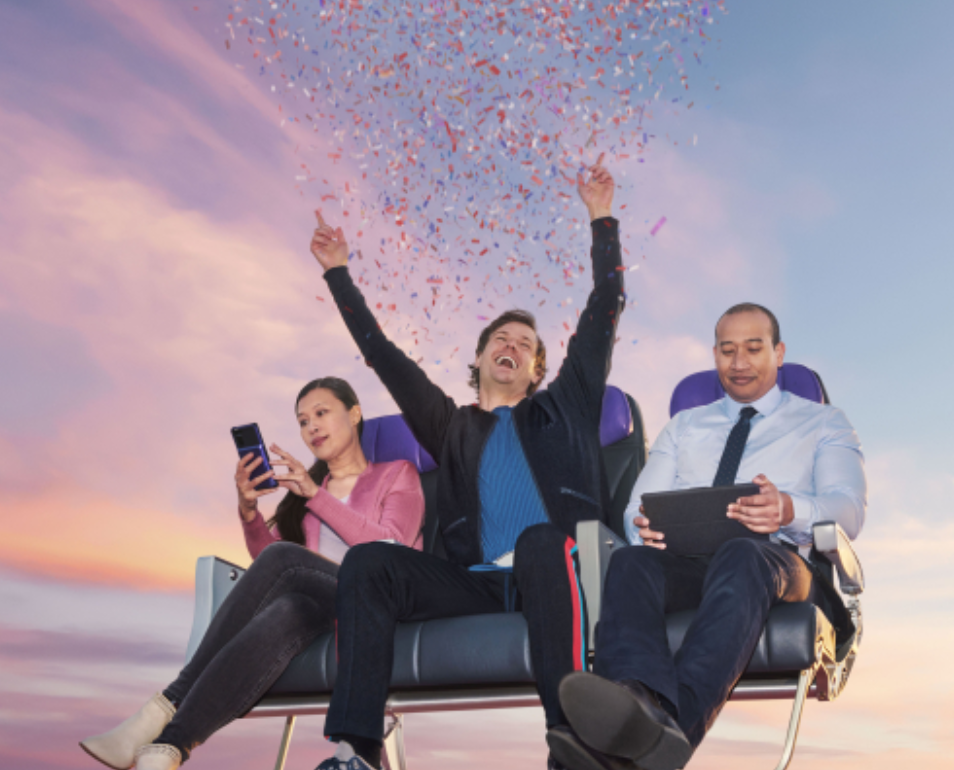
Distributed Optimization
We develop a distributed variant of the Boyle-Dykstra-Han Scheme to find the projection of a point on the intersection of several convex sets where each agent can compute projections only its associated convex set.
Collaborators: VS Borkar (IIT Bombay)
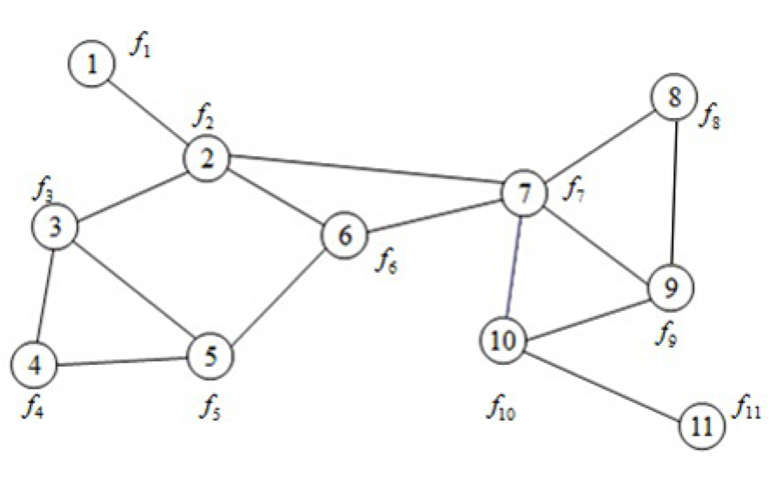